AI’s impactful role in data processing
Discover how AI and machine learning have impacted Echo's data processing workflow and ability to empower business decisions with reliable and complete geospatial datasets.
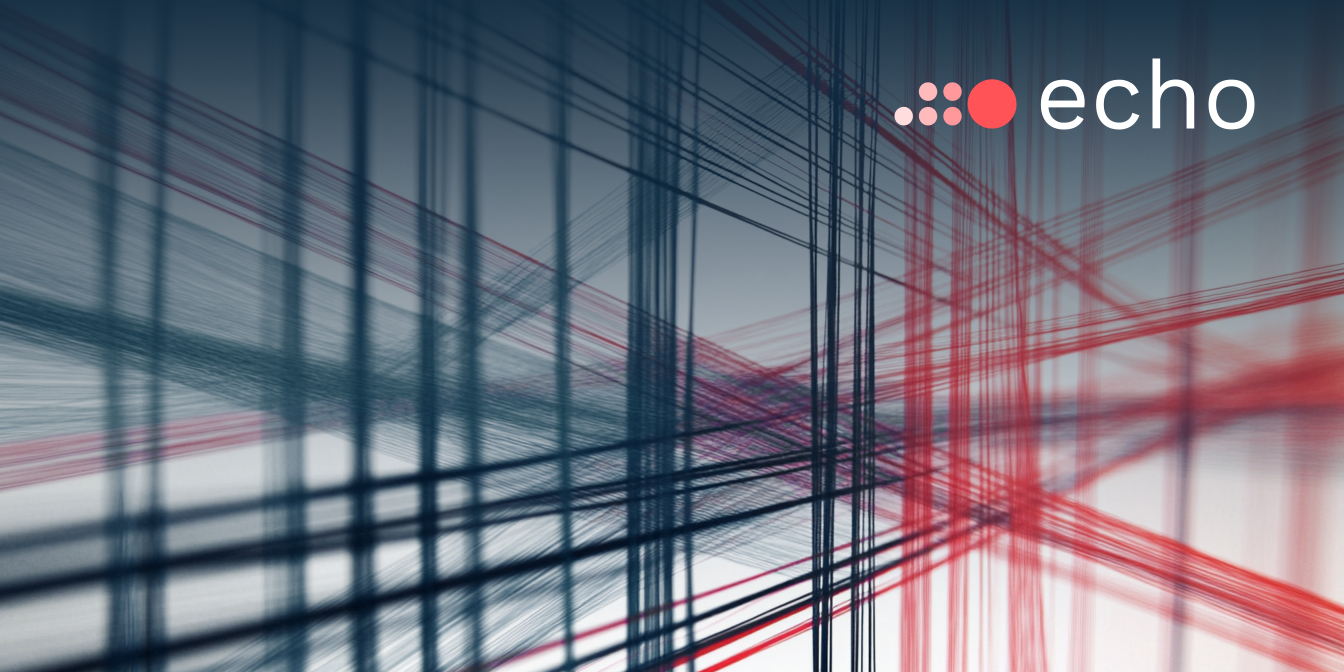
The artificial intelligence mania is difficult to ignore. Big tech firms – including Apple, Meta, Microsoft, and Alphabet – have invested substantial sums. The world’s tech space views AI as a globally transformative technology set to change the way we work and live. Whatever the conversation topic, it’s bound to come up.
Whether you buy into the hype or not, global corporate investment in AI has skyrocketed. In 2021, $276.1 billion was put into the sector by businesses worldwide and US corporations have developed 61 notable machine-learning programs. In 2022, OpenAI launched ChatGPT-3.5, a chatbot accessible to anyone with a web browser. It gained 1 million active users faster than any consumer product and now boasts 200 million monthly users. The success of ChatGPT marked a historic leap for AI.
Although there has been significant investment in recent years, artificial intelligence has been around for much longer than the last two years of hype. What we’re witnessing is the culmination of decades of research, development, and investment. Its scope is more than that of a personal assistant for writing college essays, fixing code, and generating AI images for fun. It has an impact on many facets of business.
Why is data important?
Data has always been a major component of business decision-making. It helps companies analyze and understand their clients, determine target audiences, predict needs, and generate ideas. High-volume data is invaluable for providing insights, improving decision-making, and enabling automation.
At Echo, we’re responsible for delivering geospatial data at the quality and quantity that matches the expectations of businesses who depend on its insights. With the increase demand for quality at scale, manually processing geospatial data is a taxing task. To put it simply, human effort alone is insufficient. While we are far from a Hal 9000 – and perhaps let’s keep it that way – AI allows Echo to deliver geospatial datasets that offer the quality, insight, and depth businesses expect. AI has become crucial to our data processing workflow and the way we deliver reliable and complete datasets.
AI in the data processing workflow
Artificial intelligence is a broad term encompassing the use of technology implemented in a system to reason, learn, and solve complex tasks. Machine learning is a subset of AI that automatically enables a system to learn and improve a process. It's about using algorithms to analyze large amounts of data, gain insight, and then perform a task. As an application of AI, Echo has integrated machine learning within our data processing workflow.
Tackling data duplications with machine learning
Like patio weeds, data duplications are a persistent issue that must be addressed to maintain clean datasets—just as you would treat a well-kept garden for weeds, the same is true for data. Duplicates degrade data quality and distort backend metrics, leading to unreliable insights and poor dataset quality.
During our data ingestion process, deduplication takes place in the transformation phase. After data is ingested from various sources, it undergoes multiple transformation steps to standardize, match, and enhance it—and deduplication is the first step.
These duplications can take many forms. It might be as straightforward as a repeat entry for the same point of interest (POI), or as nuanced as two POIs at the same location with varying attributes. You may encounter cases where two POIs have similar but not identical locations, sharing a brand name and category. When aggregating data from multiple sources, you might even end up with three POIs for the same place, further distorting totals if these entries aren’t properly consolidated.

If this sounds complicated, it's because it is. The variety of duplicate types makes deduplication a complex task—especially when handled manually. This is where machine learning (ML) comes in. Since we have multiple sources of data, POI duplicates will be prevalent. Through advanced modeling, the data undergoes a robust enrichment process that automates deduplication, transforming a time-consuming challenge into an efficient, streamlined workflow.
This machine-learning model is an ongoing process that needs updating and work whenever a new source of data gets added. Fine-tuning every iteration of the model is necessary to keep this automated system working smoothly.

Ongoing implementations of machine learning
Given the success of machine learning in our deduplication process, we’re expanding its use to improve our overall data ingestion workflow.
Brand matching with machine learning
To start, we’ve integrated machine learning into our brand matching process. This is a stage in the workflow where we match POIs with corresponding brands to ensure brand consistency. Clients are keen to know the brands that are associated with addresses, and we’re proud to share that our model has improved by 27%, highlighting our solution’s precision and accuracy. We’ll continue to improve, and as a next step, we plan to do the same for our category matching stage, which already has an AI-based solution.
Category matching with large language models (LLM)
Category matching is when we assign a POI to an industry, a keyword to a taxonomy. This is as simple as matching the keyword “clothing store” to the classification “retail”. It sounds straightforward but think about doing this for thousands of keywords.

Maintaining the list manually isn’t sustainable, so we’ve used LLMs to do the matching for us. This has turned out to be far more effective than traditional methods.
Translating with LLMs
LLMs have also supported us in opening our service in different countries. When expanding our country coverage, collecting POI data based solely on English terms would miss crucial information. Local language processing is essential but time-consuming given the number of languages involved. LLMs handle translations for us, preserving data quality and ensuring we don’t overlook important details.
The Future of AI-Powered Data Processing
Our machine learning models are essential to the way we deliver reliable and complete geospatial datasets. In addition to deduplication, it can fill in missing attributes and ensure every POI is associated with the correct brand. From our perspective, to meet quality demands you have to leverage technologies like AI. The power of geospatial data lies not only in its collection but in how it’s processed. Being AI-powered allows us to give businesses a critical understanding of the world around them, their customers, and market trends, empowering better decision outcomes.
FAQ
What is AI data processing?
AI data processing refers to the use of artificial intelligence to automatically clean, transform, and analyze large datasets. It helps uncover patterns, classify information, and generate insights faster and more accurately than traditional methods.
How is AI used in data processing?
AI can automate repetitive data tasks like deduplication, categorization, and anomaly detection. It also powers advanced analytics such as predictive modeling and natural language processing (NLP) for unstructured data.
What are the benefits of using AI in data pipelines?
It enhances scalability, reduces manual labor, and improves decision-making by delivering real-time insights. It enables organizations to process vast amounts of data efficiently with fewer errors.
What’s the difference between traditional and AI-based data processing?
Traditional methods rely heavily on manual rules and scripting, while AI systems learn from data patterns and improve over time. AI brings adaptability and speed to complex data environments.