Imprecisions & inaccuracies in geospatial data
Data has become a valuable tool and source for businesses executing crucial strategies, but it has its flaws. Explore how imprecisions and inaccuracies occur in geospatial data.
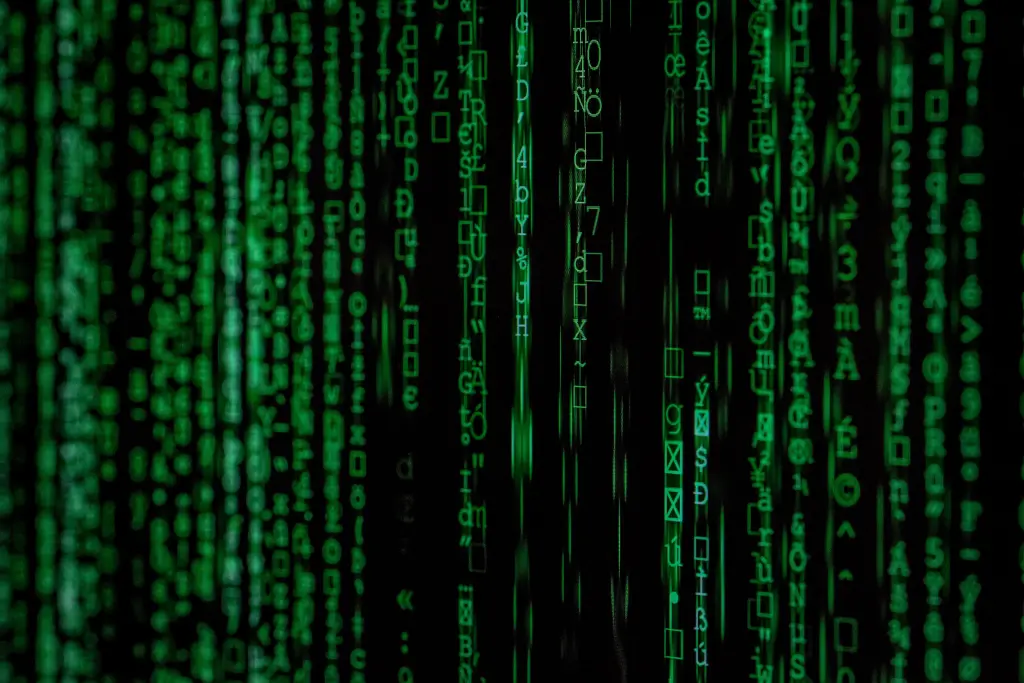
Data is alluring, it provides you with insights that can either make or break your business strategy. But it is flawed. Any amount of big data comes with its own set of inaccuracies, imprecisions, and errors. It requires an expert set of eyes to mark out those inaccuracies and to present to you with a clean, organized, and neatly labeled dataset that not only gives you the information that you need but also helps you reduce investment risks, significantly. But before we can talk about the fallacies of data, we need to understand what is meant by terms such as accuracy and precision in the geospatial sphere.
Geospatial data accuracy
Imagine you see a black coat online, it looks perfect. The right cuts, the right size, and also it assures you of the quality. Now when you order the coat it arrives in a slightly different shape. The color is quite off and it doesn’t fit you as well as you thought it would. This situation is an instance of inaccuracy. Accuracy is about how closely a dataset’s description or measurements match the real-life value. It is often measured by statistics, for example, the coat that you saw online might deliver 80% of the quality that it promised.
Geospatial data precision
Think about a bag of sugar that you are trying to weigh. You want to have precisely 2 kgs of sugar in that bag, so you put it on a weighing machine and adjust the sugar level accordingly. To ensure the measurement is correct, you put the sugar on another weighing machine and it shows that it is +/- of the expected weight. This measurement is imprecise. Precision is when you get the same measurement throughout but it doesn’t necessarily mean that it is accurate. A clock can be correct 1/1000th of a second but it might run 1 hour slow. When it comes to geospatial data, it can be said that accuracy gives you how the data is, whereas precision is the number of times it can give you that accurate data. For example, of all the polygons – which can be defined as a set of coordinates that describe a predetermined area that we create in a particular area – only 30% of those polygons are 90% accurate. This type of result is delivered with precision. As data experts, our job is to find the right balance – where 90% of the polygons are 80% accurate. That’s perfect precision. The image below would be the second figure where all the POIs are exactly in the middle. It is both precise and accurate. Accurate because its value matches that of the real world and precise because it hits the same target (same POI in this case) each time.
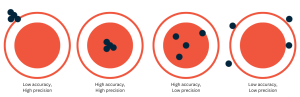
This sort of imprecision and inaccuracies are always expected in the bulk of data we source, however, we have managed to teach our algorithm – based on machine learning – to identify & fix the sources of these errors. Some of these sources can include:
Scaling for geospatial maps
Scalability becomes particularly complex as well as important because most geospatial maps are drawn on a Fractional Scale. On this type of scale, the amount of reduction between the real world and its graphic representation determines how well the GIS map can be enlarged or shrunk, if needed.
Examining this kind of scale helps us understand the problem and its effects which may vary by geographical location. We measure fractional scales by the ratio of the distance between two points on a map and the distance between those points on the ground. For example, 1 unit on the map could be 10,000 cm on the earth so the fractional scale will represent 1:10,000.
Most fractional scales are demonstrated by a display scale which includes details of a particular place as well as the size and placement of texts and symbols. The problem with this kind of display is that it can quickly become overcrowded.
This type of scale is notorious for its inaccuracies and imprecisions. The major issues of fractional scale include:
- Omitted features that are there on earth but are not represented on the map
- Representing features that do not exist on earth
- Incorrect classification of attributes
- Inaccurate location, such as the spot represented on the map might vary from the actual location on earth
- As these problems are usually expected, data experts always ensure that a firm statement of location accuracy is mentioned in a dataset. As mentioned above, 30% of polygons are 80% accurate is a statement of location accuracy. Data experts might also include statistics of uncertainty and the method of collecting the information.
Formatting bulk data
When a bulk of data comes at once from various sources, data experts always expect an immense amount of error in formatting. Such as two different sources of data can be duplicated but since they are presented differently the algorithm might read them as two separate pieces of information. If two sources provide the same phone numbers and one of those phone numbers includes an international code then it becomes necessary to first of all set a proper format. Data experts consider this type of edge case can lead to possible errors while creating a dataset.
Addressing taxonomy errors
One of the most important and common issues that data experts face is the process of classifying a huge amount of data. While classifying the data, data experts follow some standardized methods such as NAICS (North American Industry Classification System) which are established to collect, analyze, and publish statistical data.
However, companies often require data that is need-based as well as something they can rely on for their strategies. It compels data experts to ask questions like, “What data or information does the company require the most?”
Taxonomy errors occur when the data is not classified according to the company’s needs. Data experts pay close attention to taxonomy to avoid any kind of classification error that can potentially lead the dataset to be useless.
The complexity of the issues and aiming for perfection are the two things that make geospatial data analysis so demanding. Data experts like the ones at Echo Analytics take extra measures to ensure that these issues are wiped out so that the client receives accurate and precise data.
We also provide our clients with customized datasets that are classified and labeled so that they can get the information that they can rely on for their business.
FAQ
What causes inaccuracies in geospatial data?
Inaccuracies can come from GPS drift, signal obstructions, device limitations, data aggregation errors, or inconsistent POI boundaries.
How accurate is location data from mobile devices?
Mobile location data is typically accurate within 5–50 meters depending on the source: GPS is most precise, while Wi-Fi and cell-tower triangulation vary more.
Why does location data sometimes seem wrong?
Obstructions like buildings, tunnels, or weak signal conditions can misplace a device. Also, apps may report last known location instead of live data.
What’s the difference between precision and accuracy in geospatial data?
Accuracy is how close the data is to the true location. Precision refers to how consistently data is recorded — even if slightly wrong, it may still be usable if consistent.
How does Echo Analytics improve geospatial data quality?
Echo combines multiple data signals, rigorous filtering, and POI validation to reduce noise and improve the real-world relevance of geospatial insights.